Secure AI integrations
Secure AI integrations refer to the seamless incorporation of artificial intelligence (AI) technologies into existing business systems or platforms while maintaining the highest levels of security, privacy, and regulatory compliance. As AI becomes more integrated into business operations, from customer support to data analysis, ensuring the security of these integrations is critical to protecting sensitive information, maintaining operational integrity, and building trust with users and customers. Secure AI integrations help businesses leverage AI’s capabilities without exposing them to unnecessary risks such as data breaches, misuse, or unethical decision-making.
One of the key aspects of secure AI integrations is ensuring that AI systems are secure by design. This involves integrating robust cybersecurity measures from the outset of the AI deployment process. Businesses should implement end-to-end encryption to protect data as it moves between the AI system and other connected platforms, such as databases, cloud services, or third-party applications. This encryption ensures that even if data is intercepted, it remains unreadable to unauthorized parties. Furthermore, businesses should apply strong access control mechanisms, ensuring that only authorized personnel or systems have access to the AI and its associated data. Multi-factor authentication (MFA) and role-based access control (RBAC) are essential practices for minimizing the risk of unauthorized access.
Data protection and privacy are other critical considerations when integrating AI into business systems. AI technologies often rely on large datasets that can contain sensitive personal information. To protect this data, businesses must comply with data privacy regulations like the General Data Protection Regulation (GDPR), the California Consumer Privacy Act (CCPA), or industry-specific standards such as HIPAA. Secure AI integrations require careful management of customer consent, ensuring that data is only collected, processed, and stored with the explicit permission of the user. Additionally, data anonymization and tokenization techniques can help protect personally identifiable information (PII) by replacing sensitive data with non-sensitive identifiers, reducing the risk of exposure in case of a data breach.
Another important aspect of secure AI integrations is model security. AI models themselves can be vulnerable to attacks, such as adversarial attacks, where small manipulations of input data are made to trick the AI into making incorrect decisions. To protect AI systems from these types of threats, businesses should implement model validation and adversarial testing techniques. These processes involve testing the AI model with various inputs to identify weaknesses and improve its resilience against manipulation. Additionally, businesses should ensure that their AI models are regularly updated and retrained to maintain accuracy and security, especially when new threats emerge or when the underlying data changes.
Monitoring and auditing are essential practices for ensuring secure AI integrations. Once AI systems are integrated, businesses must continuously monitor their performance, security, and compliance with regulations. Automated monitoring tools can track the behavior of AI models in real-time, detecting anomalies or irregular patterns that could indicate security breaches or malicious activities. Regular security audits, including penetration testing and vulnerability assessments, help identify potential risks in the AI system before they can be exploited. Businesses should also have procedures in place for incident response, ensuring that any security breach or ethical concern is promptly addressed.
To further enhance security, API security is critical for AI integrations that involve connecting with external systems or third-party services. APIs (Application Programming Interfaces) are the bridges through which data is exchanged between systems. If APIs are not properly secured, they can become points of vulnerability, allowing unauthorized access to sensitive information. Secure AI integrations should include practices like rate limiting, authentication, and encryption to protect API endpoints and ensure that data flows securely between systems.
Ethical AI considerations are also crucial when integrating AI into business processes. Ensuring that AI systems are free from bias, discriminatory practices, and ethical concerns is important for maintaining public trust and ensuring fairness in decision-making. This involves using diverse and representative datasets to train AI models, conducting regular audits for bias, and ensuring that AI decisions can be explained and justified. Transparency in AI decision-making is essential, particularly in areas such as finance, healthcare, and law enforcement, where decisions can have significant consequences.
In conclusion, secure AI integrations require a multi-faceted approach that combines robust cybersecurity practices, data protection strategies, continuous monitoring, and ethical considerations. By focusing on securing AI systems from potential threats, protecting customer data, ensuring regulatory compliance, and maintaining transparency in AI decision-making, businesses can safely integrate AI technologies into their operations. Secure AI integrations help organizations leverage the full potential of AI while minimizing risks and maintaining the trust and safety of their customers and stakeholders.
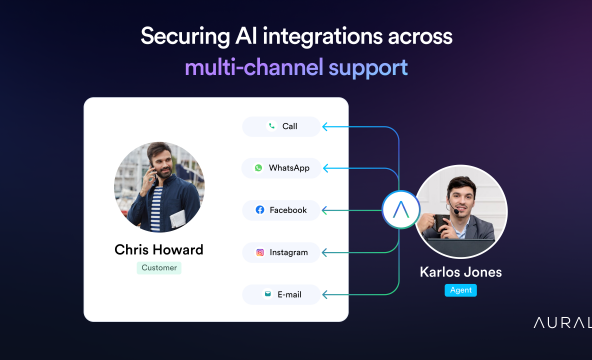
- Articles
-
Amy
- 9 min read