Secure AI deployment
Learn about secure AI deployment strategies that protect sensitive data, mitigate risks, and ensure compliance with industry regulations while enhancing performance.
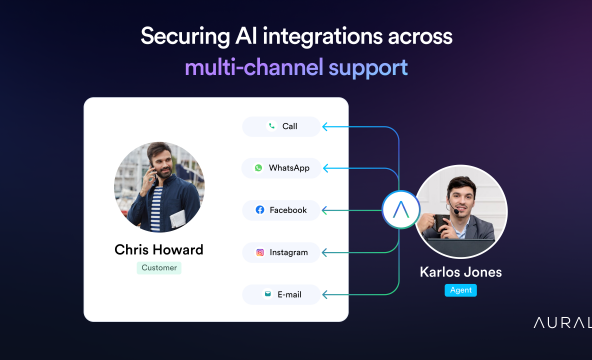
- Articles
Explore how to secure AI integrations in multi-channel support, protecting customer interactions across platforms.
-
Sid
- 9 min read