AI is helping businesses streamline their customer support for efficiency and effectiveness. While everyone’s talking about improved response times and customer satisfaction rates, did you know that AI is also helping with better decision-making in customer support?
In this post, we’re going to discuss how AI customer support tools like Auralis AI help with improved decision-making in customer support.
Table of Contents
What is decision-making in customer support and why is it important?
Decision-making in customer support typically involves determining how to handle customer queries, resolve issues, allocate resources, and optimize interactions. It requires looking into how teams can balance speed, accuracy, and personalization when engaging customers, and when an interaction requires a manual or automated resolution. Here’s why it’s important for customer support teams to dig deeper into data for decision-making:
- Improves customer satisfaction – Helps teams set up quick and accurate responses to customer queries which makes them feel heard, valued, and assisted.
- Reduces operational costs – Assists teams in reducing the time and effort spent on each customer inquiry leading to a more streamlined support process.
- Brings support efficiency – Teams can prioritize tickets, escalate issues, or automate routine queries to manage high volumes of requests without compromising quality.
- Boosts brand loyalty – Decisions that reflect customer preferences and past behaviors help foster stronger relationships.
- Enables proactive service – Businesses can anticipate customer needs and address issues before they arise, reducing friction and improving the overall experience.
Ways in which AI helps with decision-making in customer support
Customer support solutions like Auralis AI are helping businesses with better decision-making by offering data-driven insights in real time. Here are some of the ways in which AI assists in making informed decisions in customer support teams:
1. Data-driven insights and analytics
AI can analyze vast amounts of customer data including current and past interaction, browsing behavior and sentiments. This provides businesses with insights that help customer support teams determine the best response to specific queries and identify patterns in recurring issues.
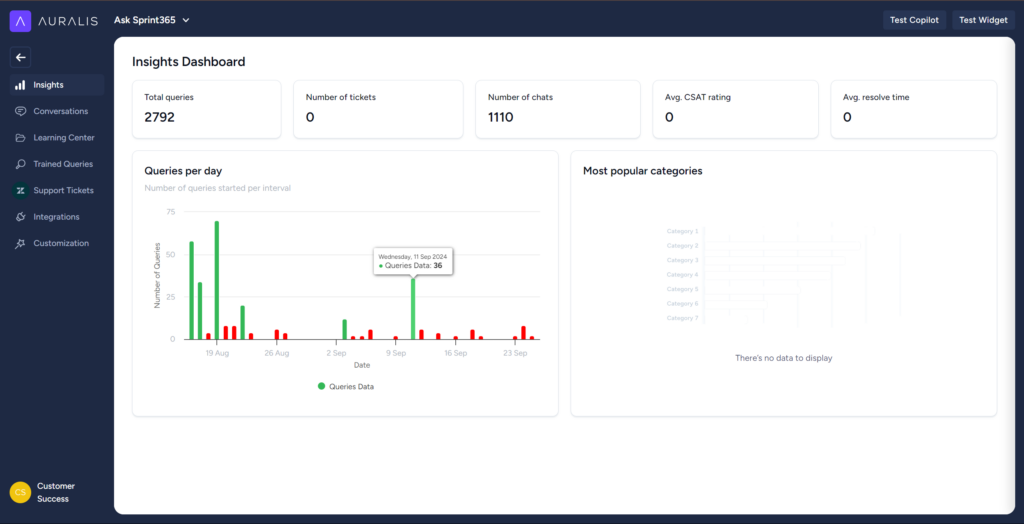
2. Real-time assistance and recommendations
AI-powered customer support solutions can assist agents in real-time. This could include providing recommended responses or troubleshooting steps based on the context of the conversation. They could also pull up relevant information like FAQs, previous purchase history or suggested solutions, allowing the agent to deliver a quick and accurate response.
3. Automated ticket routing
AI can help with automatically categorizing and routing customer inquiries to the appropriate department, teams, or agents. This could be based on the nature of the query or the complexity of the issue, ensuring that it gets handled by the most qualified person. Businesses can drastically reduce the response time and improve support efficiency with this decision-making in customer support.
4. Predictive analytics for proactive support
AI can analyze past interactions more accurately to predict future behavior or issues. This includes being able to identify customers who are at risk of churning due to unresolved issues or unsatisfactory experiences. The insights help customer support teams create strategies that use forecasting to optimize efforts.
5. Sentiment analysis for contextual understanding
So far, understanding customer sentiment has been based on the inputted feedback and reviews on customer support or an individual understanding of the conversation. AI tools remove the guesswork and assumptions from the equation to better analyze sentiments – be it frustration, satisfaction, or confusion.
6. Multi-lingual support for markets
AI customer support tools can help businesses offer customer support and services in multiple languages. But in addition, they can also help them understand which demographics tend to seek the most amount of support and the nature of queries. This helps businesses better prepare for each target location, tailoring their resources according to the audience segments.
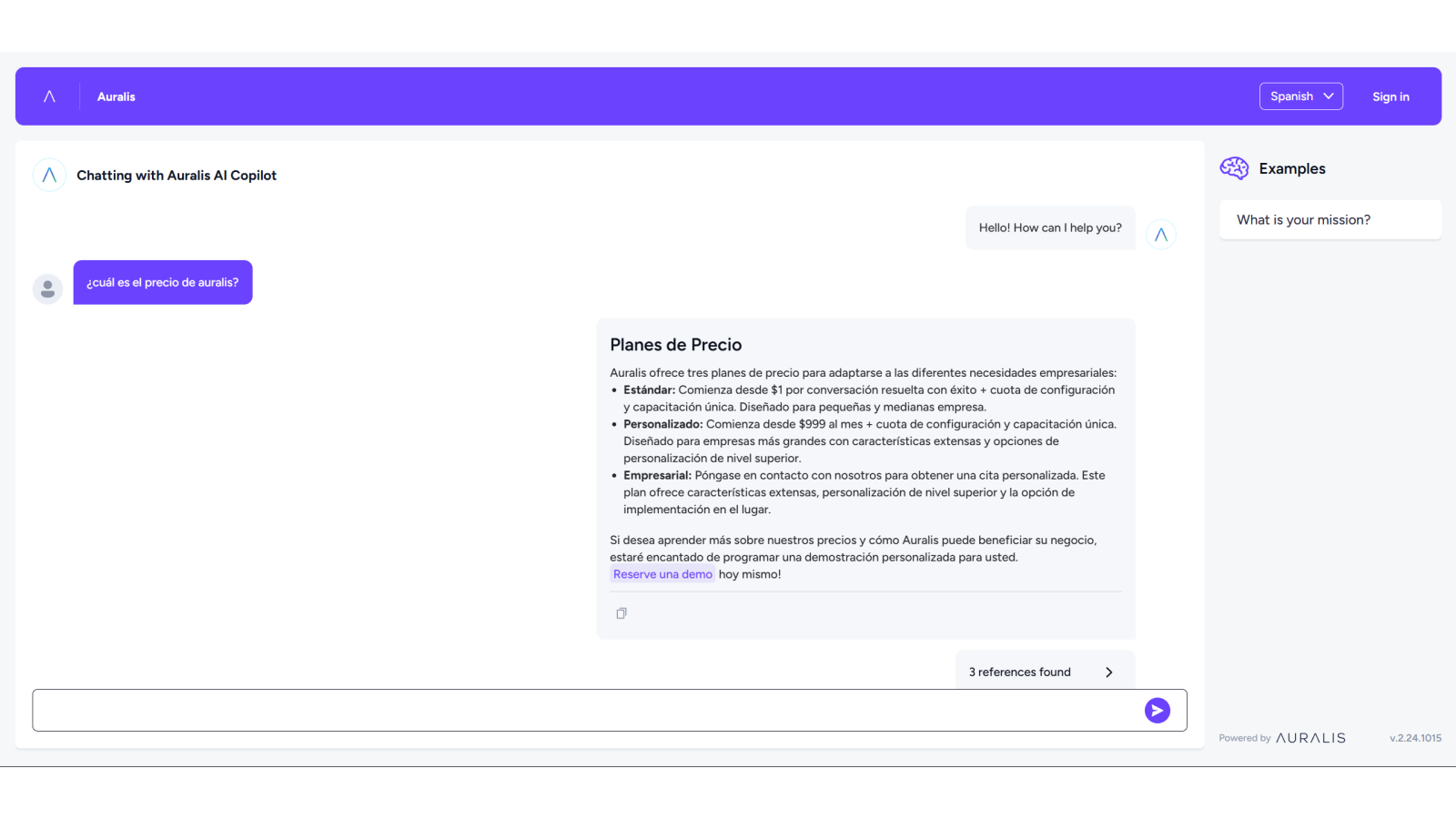
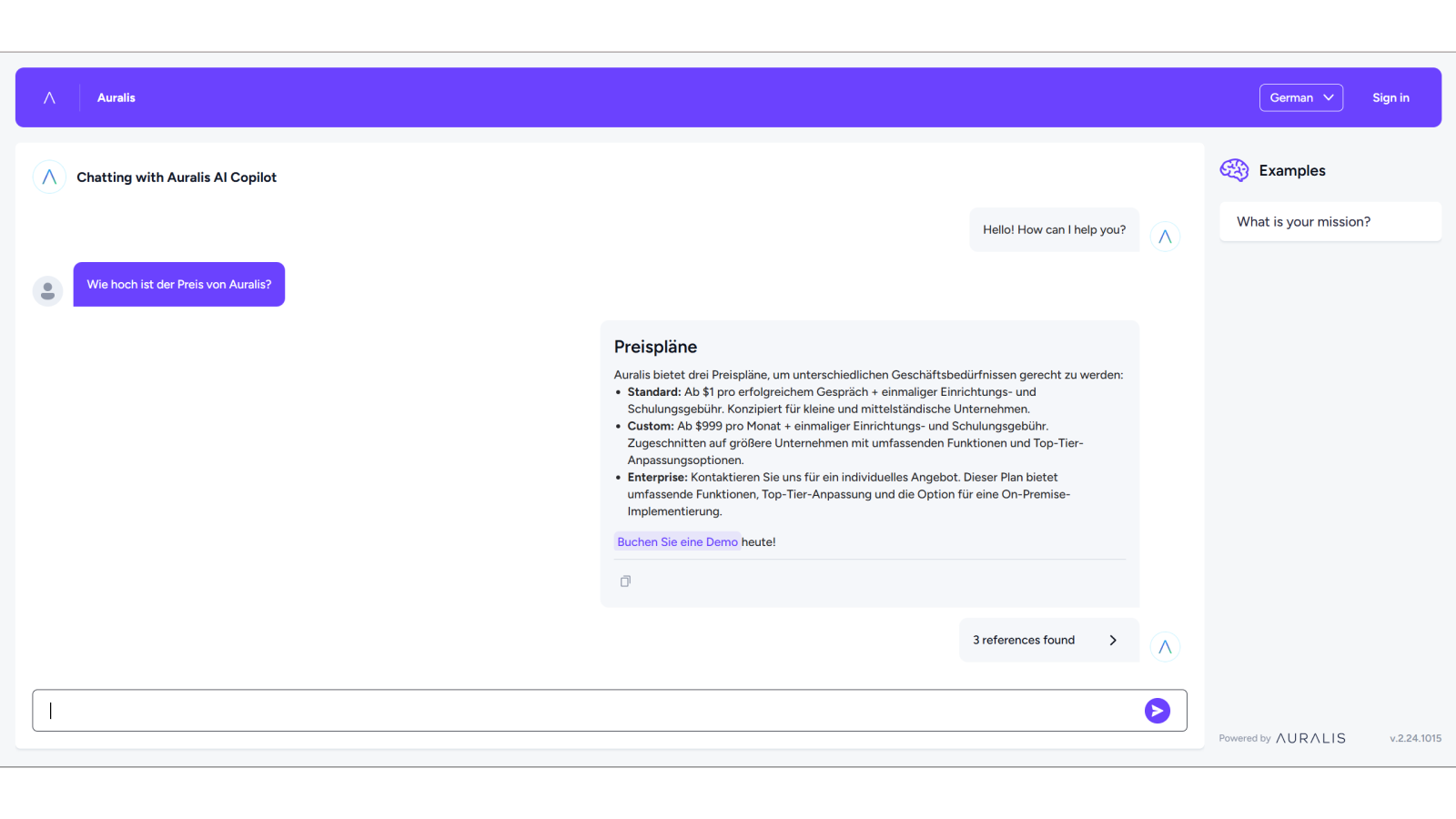
Examples of AI-driven decision-making in customer support
Let’s put the above into perspective:
Example 1 – Real-time product support in e-commerce
Online stores receive hundreds of customer queries around product availability, size recommendations and order tracking. With AI chatbots, brands can access real-time data to answer these questions automatically. But if the query is more complex – such as a delayed order or product quality issue – the AI system can decide to prioritize the ticket and trigger a hand-off to a human agent.
Example 2 – Predictive customer retention in SaaS
With the increasing competition in SaaS, customer churn is becoming a common challenge. When using AI in customer support, companies can identify patterns that can lead to a drop-off/ churn. For example, customers opening multiple support tickets related to onboarding and setup are at a higher risk of canceling their subscriptions. This helps the team decide on following up with additional resources or a personalized demos in a timely manner.
Example 3 – Personalized post-purchase engagement for retailers
Based on customer preferences and past purchase behavior, retail companies can send personalized product recommendations, exclusive offers, or even content to keep customers engaged. This can help the company upsell and cross-sell even through support using AI chatbots that can access information from the CRM.
Best practices for implementing AI in customer support decision-making
AI-powered solutions can give you access to a lot of information on your customers for better decision-making. But to make sense of that information, here are some best practices to follow:
- Start with a clear objective – Define what you want to accomplish with AI in customer support decision-making. Are you looking for ways and opportunities to improve response times, improve personalization, reduce cost of customer support, or scale the department to support other growth units?
- Train AI with quality data – Ensure the input you provide to AI is accurate or up-to-date; irrespective of the format it is available in. The more accurate a response is, the better is the interaction with the customer, leading to improved insights on them.
- Ensure seamless human handoff – Ensure that making the handoff between AI chatbots and human agents is seamless with the insights you gather from the solution. The setup should have a clear escalation path, complete with relevant customer data to prevent high transition times.
- Prioritize transparency – As you scale using AI for customer support, be transparent with customers. Let them know they’re interacting with a bot and provide options to speak with a human agent.
- Seek intelligent solutions – Decision-making with AI is only simple if the customer support solution you use can provide actionable insights. For example, apart from the general analytics dashboard, Auralis AI also summarizes all queries into actionable insights.
Conclusion
AI is changing how businesses approach decision-making in customer support.
By automating routine tasks, providing real-time insights, and leveraging predictive analytics, AI is helping support teams to make faster, more informed decisions to improve customer experiences. And leading businesses across industries can be seen embracing this!
Want to know how AI can help with decision-making in customer support?